what does chemo do to the intestines over 6 months period
- Brusk report
- Open Access
- Published:
Effect of chemotherapy on the microbiota and metabolome of human being milk, a example report
Microbiome volume 2, Article number:24 (2014) Cite this article
Abstract
Background
Homo milk is an important source of bacteria for the developing infant and has been shown to influence the bacterial composition of the neonatal gut, which in turn tin affect disease take chances subsequently in life. Human milk is as well an important source of nutrients, influencing bacterial composition but also directly affecting the host. While recent studies accept emphasized the adverse effects of antibody therapy on the infant microbiota, the effects of maternal chemotherapy have non been previously studied. Hither nosotros report the furnishings of drug administration on the microbiota and metabolome of human milk.
Methods
Mature milk was collected every two weeks over a 4 calendar month catamenia from a lactating woman undergoing chemotherapy for Hodgkin's lymphoma. Mature milk was besides collected from healthy lactating women for comparison. Microbial profiles were analyzed by 16S sequencing and the metabolome by gas chromatography–mass spectrometry.
Findings
Chemotherapy acquired a pregnant divergence from a healthy microbial and metabolomic profile, with depletion of genera Bifidobacterium, Eubacterium, Staphylococcus and Cloacibacterium in favor of Acinetobacter, Xanthomonadaceae and Stenotrophomonas. The metabolites docosahexaenoic acid and inositol known for their beneficial effects were also decreased.
Decision
With milk contents beingness critical for shaping baby immunity and development, consideration needs to be given to the impact of drugs administered to the mother and the long-term potential consequences for the health of the infant.
Background
Colonization of the neonatal gut plays a pivotal function in gastrointestinal, immunological and neurological development, with 1 of the initial sources of bacteria being the mother'southward milk [1, two]. Breastfed infants have been shown to have lower incidences of asthma, diarrhea, and necrotizing enterocolitis compared with formula-fed infants [3]. This protective effect may exist due, in part, to the types of leaner present in milk, as infants fed formula supplemented with probiotics were better protected against these conditions compared to those just fed formula [four–6]. The leaner acquired during infancy tin can influence affliction risk after in life and play a major office in determining the future composition of the developed microbiome [5]. Thus, factors that bear on the milk microbiota accept important health consequences for the child not simply during development just also into adulthood. In addition to the microbiota, the metabolites of human being milk, such as fatty acids, carbohydrates, proteins and vitamins, also play an of import role in babe evolution and long-term health [7–10].
Mail service-delivery, many women are prescribed pharmaceutical agents for various reasons. While most over-the-counter drugs and antibiotics are non contraindicated during breast feeding [eleven, 12], when it comes to chemotherapeutics, the recommendation is that breastfeeding should be avoided until the drug has been cleared from the milk [13]. In a case report of a 70 mg infusion of cisplatin, no detectable levels were institute in milk after 66 hours [14], and in another case written report using doxorubicin (trade name Adriamycin), no detectable levels were seen after 72 hours [15]. In our particular study, the field of study was brash that breastfeeding could resume 12 days after each chemotherapy session.Here we present the outset report on the effects of chemotherapy on microbial and metabolomic profiles in human being milk over a 4-month menstruation in a breastfeeding woman undergoing handling for Hodgkin's lymphoma (Figure 1).
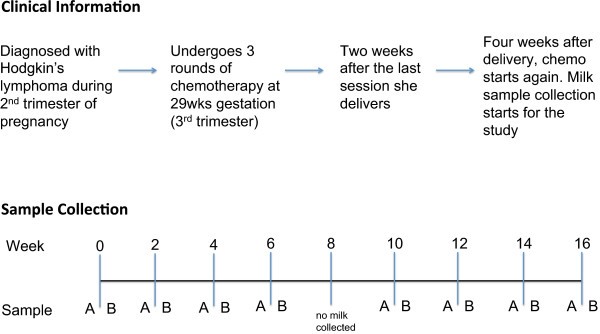
Summary of clinical information and sample collection. Milk samples were collected from a lactating woman undergoing chemotherapy for Hodgkin's lymphoma. Milk samples were nerveless every 2 weeks over a four-month menses. At each session milk was nerveless fifteen to 30 minutes earlier (sample A) and after (sample B) chemotherapy. The duration of chemotherapy treatment was 2 hours. No milk was nerveless at calendar week viii due to scheduling conflicts.
Methods
Clinical samples and written report pattern
Upstanding approving was obtained from Western Research Ethics Board and Lawson Health Research Institute, London, Ontario, Canada. Subjects provided written consent for sample drove and subsequent analyses.
Milk collection and processing
Mature milk was collected from a lactating woman undergoing the ABVD chemotherapy authorities (Adriamycin (twoscore mg), Bleomycin (xvi units), Vinblastine (nine.6 mg), Dacarbazine (600 mg) for Hodgkin's lymphoma at the London Wellness Sciences Center, London, Ontario. Mature milk was also nerveless from 8 healthy women recruited from London, Ontario and the surrounding area. Wearing sterile gloves the women cleaned their nipple and surrounding area with sterile saline swabs to reduce the presence of skin bacteria. Milk was collected using a sterile HygieniKit Milk Collection Organization (Ameda, Buffalo Grove, IL, Usa) fastened to an electric breast pump. Between 5 and fifteen ml of milk was pumped into a sterile tube and kept on ice until transfer to the laboratory, which occurred within i hour of collection. Samples were aliquoted and stored at -20°C until DNA extraction.
DNA isolation
After thawing on ice, 2 ml of milk were spun down at thirteen,0001000 for x minutes and the supernatant discarded. The pellet was and then homogenized in 1.4 ml of ASL buffer (QIAamp® DNA Stool Kit, QIAGEN: Valencia, CA, Us) and 400 mg of 0.ane mm diameter zirconium-drinking glass chaplet (BioSpec Products, Bartlesville, OK, USA). Mechanical and chemic lyses were performed by bead beading at 4,800 rpm for 60 due south, then 60 s on ice (repeated twice) using a mini-beadbeater-one (BioSpec Products) and then incubated at 95°C for five minutes. Subsequent procedures were performed using the QIAGEN QIAamp® Dna Stool Kit according to the manufacturer's protocol, with the exception of the last step in which the column was eluted with 120 μl of elution buffer. DNA was stored at -twenty°C until further use.
V6 16S rRNA cistron sequencing
PCR distension
The genomic DNA isolated from the clinical samples was amplified using the barcoded primers V6-LT: 5′CCATCTCATCCCTGCGTGTCTCCGACTCAGNNNNNCWACGCGARGAACCTTACC3′ and V6-RT: v′ CCTCTCTATGGGCAGTCGGTGATACRACACGAGCTGACGAC3′, which amplify the V6 hypervariable region of the 16S rRNA gene. The PCR was carried out in a forty μl reaction containing 5 μl of DNA template (or nuclease-free h2o as a negative command), 1.v mM MgClii, 0.eight μM of each primer, 4 μl of ten× PCR Buffer (Invitrogen, Burlington, ON, Canada), 0.2 mM dNTPs, 0.05U Taq Polymerase (Invitrogen) and 0.15 μg/μl bovine serum albumin. Thermal cycling was carried out in an Eppendorf Mastercyler nether the following weather: initial denaturation at 95°C for 2 minutes followed by 25 cycles of 95°C for 1 minute, 55°C for one minute and 72°C for ane minute. After amplification, the Deoxyribonucleic acid concentration was measured with the Qubit® two.0 Fluorometer (Invitrogen) using the broad range analysis. Equimolar amounts of each PCR production were pooled together and purified using the QIAquick PCR purification kit (QIAGEN). The PCR purified sample was then sent to the London Regional Genomics Eye, London, Ontario, Canada for V6 16S rRNA gene sequencing using the Ion Torrent platform as per the eye's standard operating process.
Sequence processing and taxonomic consignment
Custom Perl and Bash scripts were used to de-multiplex the reads and assign barcoded reads to private samples. Reads were kept if the sequence included a perfect lucifer to the barcode and the V6 16S rRNA factor primers. Reads were clustered by 97% identity into operational taxonomic units (OTUs) using UClust v.3.0.617 [16]. OTUs that represented ≥1% of the reads in at least one sample were kept, while those that did not run into the cutoff were discarded. Taxonomic assignments for each OTU were fabricated past the Ribosomal Database Project (RDP) SeqMatch tool [17]. From the elevation twenty matches to the RDP named isolates database, the full taxonomy was retained for matches with the highest S_ab score. For multiple top matches with equal scores, the highest common taxonomy was retained (for example, genus level if multiple species matched as well). Since the maximum number of matches displayed per sequence is 20, the RDP taxonomic assignments were verified by BLAST confronting the Greengenes named isolates database with an output of 100 hits [18]. Taxonomy was assigned based on hits with the highest percentage identities and coverage. If multiple hits fulfilled this criterion, classification was re-assigned to a higher mutual taxonomy. In instances where the highest percentage identity/coverage yielded a unmarried lucifer, if this were <90% and the S_ab score from RDP was <0.vii, taxonomy was assigned at the family level instead of at the genus level. A summary of each OTU classification and its sequence is shown in Additional file i. The raw sequencing reads generated in this report have been deposited to the NCBI Short Read Archive (SRA) database [SRA:SRP041626].
Data analysis
Weighted UniFrac distances were calculated in QIIME [19] by using a phylogenetic tree of OTU sequences built with FastTree [twenty] and based on an OTU sequence alignment with MUSCLE [21]. The QIIME pipeline was likewise used to summate Shannon's variety index (logarithms with base 2) and to generate principal coordinate analysis (PCoA) plots. Weighted UniFrac distances compare microbial profiles (presence/absenteeism and affluence) between samples (i.due east., beta-diversity) [22] while Shannon's diversity index evaluates the microbial diversity within a sample (i.e., alpha variety). The college the Shannon's variety index, the more diverse a sample is and a value of zero indicates the presence of only ane species [23]. PCoA plots let ane to visualize the UniFrac distance matrix and plot the values on a set of orthogonal axes that capture the greatest amount of variation between all samples tested. For beta-diversity analyses, the data set was rarified to the everyman read count/sample, which was 734 reads. A summary of clinical data, including total number of sequence reads per sample, is shown in Additional file 2. Barplots, boxplots and stripcharts were all generated in R [24].
Statistical analysis
The ALDEx R package version 2 [25] was used to compare genera between the non-treatment and chemotherapy handling groups (as portrayed in the boxplots). Microbiome data represent proportional distributions and are thus not independent of each other. This ways that a decrease in one organism volition inevitably pb to a concomitant increase in another organism. For example, if a sample has two organisms A (l%) and B (50%) and A is completely killed by an antibiotic, the proportion of B in that sample will now exist 100% even if its actual abundance has not changed. The ALDEx R parcel estimates the technical variation inherent in loftier-throughput sequencing by Monte-Carlo sampling from a Dirichlet distribution [26]. The Monte-Carlo replicates are transformed using the centered log-ratio transformation, which takes the logarithm of the Monte-Carlo estimates of organism abundances in each sample divided past the per-sample geometric mean organism abundance [27]. This transformation has several desirable properties that exercise not exist in proportional information, notably subcomposition coherence and linear sample independence. Data transformed in this fashion permit the use of standard statistical tests to decide significance. Values reported in this manuscript represent the expected values of 128 Dirichlet Monte-Carlo instances. A value of zero indicates that organism abundance is equal to the geometric mean affluence. Thus, organisms more abundant than the mean will have positive values and those less abundant than the hateful will take negative values. Base two was used for the logarithm and so differences between values represent fold changes. Statistical significance for these comparisons was determined by a Mann-Whitney U test with P < 0.05 and a fake discovery charge per unit (FDR) of < 0.1 using the q values output by the fdrtool R package [24].
The unpaired Student's t-exam was used to compare Shannon'south diversity index (P < 0.05).
Sample training gas chromatography-mass spectrometry
To extract metabolites, 100 μl of milk were mixed with 400 μl pure methanol. Samples were vortexed for fifteen south and centrifuged for ten minutes at 9,000grand. Supernatants (200 μl) were transferred to gas chromatography-mass spectrometry (GC-MS) vials and ii.5 μl of ribitol solution (2 mg/ml) was added to each vial as an internal standard. Samples were dried to completeness using a SpeedVac. Afterwards drying, 100 μl of two% methoxyamine•HCl in pyridine (MOX) was added to each sample for derivitization and samples were incubated at l°C for ninety minutes. North-methyl-N-(trimethylsilyl) trifluoroacetamide (100 μl) was then added to each vial and incubated at fifty°C for 30 minutes. Samples were transferred to microinserts earlier running on GC-MS (Agilent 7890A GC, 5975 inert MSD with triple centrality detector, 30 m DB5-MS column with 10 chiliad duraguard column). Samples were run using 1 μl injections on browse mode and a solvent delay of 10 minutes. Run time was threescore minutes per sample. Each sample was run twice non-consecutively to ensure consistency throughout the sequence.
GC-MS data assay
Chromatogram files were converted to ELU format using the AMDIS Mass Spectrometry software [28]. Chromatograms were aligned and abundance of metabolites calculated using the Spectconnect software [29] with the support threshold set to depression. In gild to determine if differences between week 0 and chemotherapy (weeks two to sixteen) existed, principle component assay (PCA) was conducted in SIMCA (Umetrics, San Jose, CA, USA) using the relative affluence matrix output from Spectconnect. Data were mean centered and pareto scaled prior to PCA. Contained unpaired t-tests with Bonferroni correction were calculated in Excel to determine metabolites that were significantly contradistinct past chemotherapy (P < 0.05). Compounds that besides contributed to the separation of week 0 from chemotherapy samples co-ordinate to the PCA loadings plot (compounds in bottom left quadrant) were chosen for further investigation.
Findings
Chemotherapy affected both bacterial diversity and bacterial profiles in human being milk. Bacterial diversity within samples was lower in milk collected throughout chemotherapy compared with milk samples collected at week 0 and from healthy lactating women (Figure 2). Bacterial profiles at calendar week 0 were similar to those from good for you women, although this changed within two weeks of treatment (Figure 3A). Samples collected at weeks 4 to 16 shared similar profiles and differed from week two and from week 0/good for you samples (Figure 3A). These differences were non due to natural changes over fourth dimension, equally the bacterial community in two milk samples analyzed from a healthy field of study did not change over a 4-month menstruation (Figure 3A, green samples). The bar plot in Figure 3B shows the bacterial communities present in these samples with a striking increase in abundances of Acinetobacter and Xanthomonadaceae in milk collected during chemotherapy. A comparison of relative abundances of Acinetobacter, Xanthomonadaceae and Stenotrophomonas (a genus belonging to the Xanthomonadaceae family) betwixt the chemotherapy (weeks 4 to sixteen) and non-treatment (calendar week 0 and healthy samples) groups is displayed in Figure iv and were significantly higher during chemotherapy. We as well examined the differences between three bacteria believed to confer benign wellness effects to the baby, Bifidobacterium, Eubacterium and Lactobacillus. The offset 2 were significantly decreased during chemotherapy whereas no differences were observed for Lactobacillus (Effigy 4). Overall, a full of 22 out of the 49 genera identified were differentially abundant between the 2 groups (Additional file three). While the core microbiome (that is, taxa that were nowadays in 100% of the samples) was somewhat similar between the 2 groups, it is interesting to note that Stenotrophomonas was nowadays in every chemotherapy sample and Lactobacillus and Eubacterium were present in every good for you and calendar week 0 sample (Additional file 4).
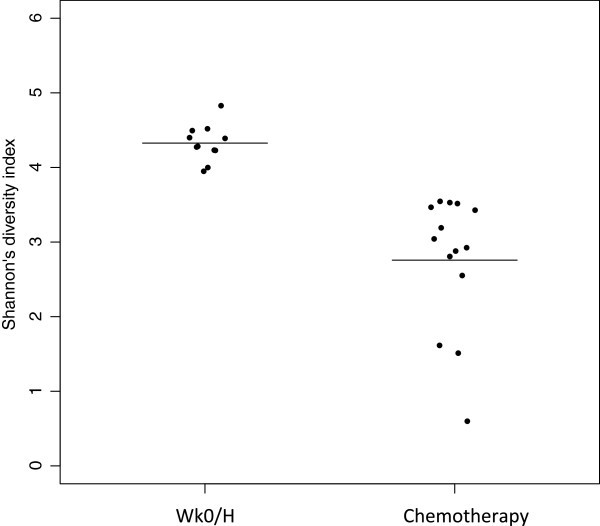
Changes in bacterial variety as a result of chemotherapy. Bacterial diversity within a sample (i.due east. alpha variety) was measured by calculating Shannon'south diversity index. Each bespeak on the graph represents a subject area with the line representing the mean for all samples within a group. The college the index the greater the bacterial diversity found within a sample. The hateful of the 'Wk0/H' grouping (week 0 and healthy samples) was 4.three, and that of the chemotherapy group (weeks 2 to 16) was two.8. Groups were statistically dissimilar from each other every bit measured by unpaired Pupil'south t-test (P < 0.05).
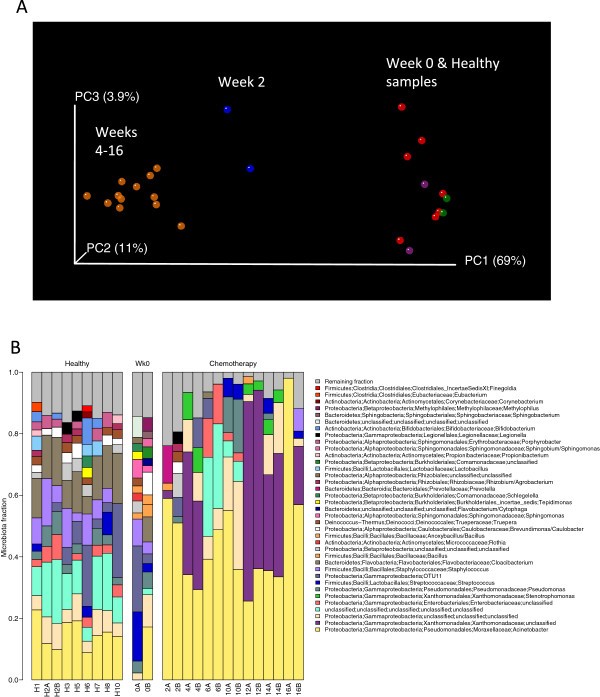
16S rRNA sequencing analysis of bacteria in human milk. Milk samples were collected from a lactating woman undergoing chemotherapy as described in Figure 1 as well as from viii healthy lactating women. (A) Weighted UniFrac PCoA plot. Each milk sample, represented by a coloured circle, is plotted on this three-dimensional, three-centrality plane representing 84% of the variation observed betwixt all samples. Samples that cluster together are similar in biota composition and abundance. Orange circles represent samples collected from weeks 4 to 16 of chemotherapy, bluish circles represent samples nerveless at week ii of chemotherapy, purple circles stand for samples nerveless at week 0, blood-red circles correspond milk samples from healthy lactating women (only one time signal) and green circles correspond milk samples from a healthy lactating women collected 4-months apart. Every bit shown past the plot, there were three distinct groups: (i) week 0 samples and salubrious milk samples; (ii) calendar week two of chemotherapy; and (iii) weeks 4 to xvi of chemotherapy. Data were rarified to 735 reads/sample. (B) Barplot showing the relative abundances of different genera in each sample. Each bar represents a bailiwick and each coloured box a different genus. The height of the coloured boxes represents the relative abundance of that genus inside the sample. Genera that were less than ii% abundant in a given sample were placed in the 'Remaining fraction' at the acme of the graph (grey boxes).
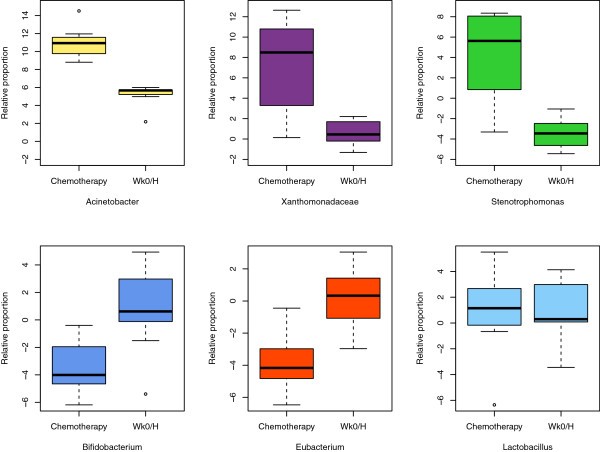
Comparison of relative proportions of bacterial taxa betwixt treatments. Boxplots comparing vi bacterial taxa between samples collected during chemotherapy (weeks 4 to 16) and those without treatment (week 0 and healthy samples (Wk0/H)). The box signifies the 75% (upper) and 25% (lower) quartiles and thus shows where 50% of the samples lie. The black line within the box represents the median. The whiskers stand for the lowest datum still within one.5 interquartile range (IQR) of the lower quartile and the highest datum still within 1.5 IQR of the upper quartile. Outliers are shown with open circles. The value '0' represents the geometric hateful abundance; thus, values higher up 0 are more arable and values less than 0 are less abundant than the geometric mean. Significant differences were observed between the two groups for all taxa graphed (Mann-Whitney U test P < 0.05, FDR <0.one).
The metabolic profile also changed as a result of chemotherapy (Figure 5) and was similar between weeks 2 and xvi, but dissimilar to that observed at calendar week 0. A total of 226 metabolites were detected past our GC-MS method, 12 of which were significantly different between the week 0 and chemotherapy (weeks 2 to xvi) groups (Table 1). Additional file 5 shows the relative abundances of all metabolites detected in milk and stripcharts in Additional file six testify the distribution of key metabolites detected between the two groups.
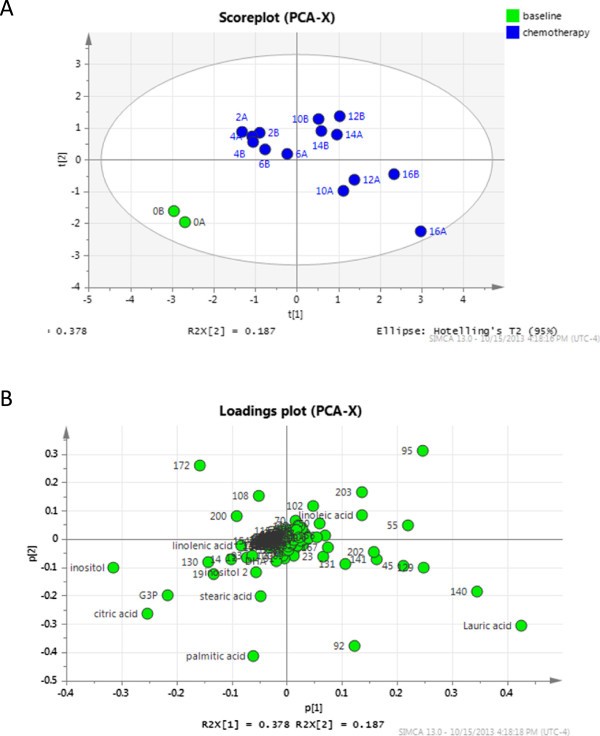
Principle component analysis of metabolites in breast milk at calendar week 0 and during chemotherapy. (A) Scoreplot displaying the distribution of samples based on metabolites alone, where the distance between samples represents how similar the metabolome of those samples are. Each point represents the boilerplate of ii technical replicates. (B) Loadings plot. Each point represents a metabolite. Metabolites present in a given quadrant of the loadings plot are nowadays in highest abundance in samples present in the same quadrant of the scoreplot (A).
Discussion
This study shows that a course of chemotherapy has significant effects on bacterial and metabolic profiles in homo milk, moving away from those of salubrious lactating women. Of note, the subject field did non report any additional drug use, antibiotics, illness or major changes in nutrition over the course of the study.
The consequences of decreased bacterial diversity in human being milk and the implications on the child are still unknown; however, the decreased milk variety could impact intestinal diversity and information technology has been shown that low intestinal diversity in the first weeks of life is associated with necrotizing enterocolitis [30] and an increased risk of allergy and atopy in school-historic period children [31]. Lower intestinal diversity has also been observed in children with type 1 diabetes compared to age-matched controls [32].
In addition to overall changes in microbial profiles, we observed a significant subtract in the relative abundance of Bifidobacterium in the chemotherapy group compared to the non-handling group. Bifidobacterium is the predominant organism in the gut of breastfed infants, attributed to its ability to metabolize the human being milk oligosaccharides present in large amounts in milk [33, 34]. Maternal levels can too affect gut Bifidobacterium affluence, with low levels in milk correlating with low levels in the neonatal gut [35]. The potential consequences of decreased numbers of Bifidobacterium being passed on from the female parent to the neonate could exist an increased risk of asthma or obesity later in life. Loftier levels of Bacteroides have been reported in the gut of infants with low levels of Bifidobacterium[36] and early colonization with high Bacteroides counts has been associated with an increased adventure of developing asthma and obesity [37–39]. In addition, depleted levels of Bifidobacterium have been shown to promote colonization of opportunistic pathogens such as Klebsiella and Citrobacter[36].
Staphylococcus, including coagulase negative species, are one of the predominant organisms in human milk [40–42], and were also significantly reduced as a upshot of chemotherapy. Information technology has been shown that numerous human milk isolates of Staphylococcus epidermidis can inhibit the growth of Staphylococcus aureus[43], the main causative amanuensis of mastitis, which is a painful inflammatory condition of the breast that often leads to premature abeyance of breastfeeding in many women. While we were non able to identify the Staphylococcus in our samples downward to the species level with 16S rRNA factor sequencing, culture analysis on mannitol salt agar plates did bear witness that the Staphylococcus isolates were not Southward. aureus and the select few that were tested were coagulase negative. This reduction of Staphylococcus (likely coagulase negative species) as a issue of chemotherapy could brand lactating women more than prone to infections, affecting both themselves and their infants. Like Bifidobacterium, Staphylococcus is passed from the milk to the neonate, with college numbers in the intestine of breastfed compared to formula-fed infants [one]. Interestingly, a metagenome analysis revealed the presence of immunosuppressive motifs in bacterial Dna from man milk, with the majority of these belonging to Staphylococcus[41]. The exposure of the neonate to this Dna, either ingested from the milk or through live bacteria that have released their DNA once in the gut, could help to regulate the infant'southward immune response against a variety of innocuous bacterial, environmental and food antigens.
The utilization of bacterial products by other bacteria is termed metabolic cross-feeding and plays an of import office in bacterial selection. For instance, the byproducts of bacterial metabolism, such every bit lactate and acetate production, are utilized as an energy source by many butyrate-producing leaner [44–47] such equally Eubacterium, which was decreased in our chemotherapy group. Butyrate is important for health, as information technology reduces inflammation and metabolic diseases, promotes colonic repair and protects confronting colon cancer [48, 49]. On the other hand, some pathogens persist and crusade disease only in the presence of certain commensal bacteria [50], likely due to the metabolites produced. Thus, changes in bacterial communities in human milk volition inevitably alter the metabolic milieu, selecting for bacteria able to utilize those metabolites. Equally a result, potential shifts from a healthy and balanced intestinal microbiota can occur, having important consequences on health.
Docosahexaenoic acid (DHA), inositol and an unknown polyunsaturated fatty acid were amongst the 12 metabolites that differed between calendar week 0 and weeks 2 to xvi, with reduced levels detected during chemotherapy (weeks two to 16). DHA is the near abundant long chain polyunsaturated fatty acid in the brain, retina and nerve cells and is supplied mainly through breast milk [51]. DHA deficiencies lead to reduced brain, heart and neuronal development [51] and it has been observed that breastfed infants take better visual acuity and neuronal development compared to those fed formula [52]. Reduced levels of DHA and alpha-linolenic acid (a precursor of DHA) accept been reported in milk of mothers with atopic children compared to milk from mothers with not-atopic children [53, 54]. Another chief metabolite in the neonatal brain is inositol, which is important for osmoregulation, cellular diet and detoxification [55]. Palmitic acid levels were as well reduced during chemotherapy, though the results were not pregnant. Palmitic acid is the most abundant lipid in human milk and has been shown to increment bone strength in infants [56] and limit abdominal damage and pro-inflammatory immune responses in mice [57]. While changes in metabolite concentrations do occur over the course of lactation, especially between colostrum and mature milk, no changes in the above metabolites over the course of the first year of life have been observed in mature milk [58–61]. Due to the high variability in milk metabolites between individuals, we did not have plenty samples from healthy women to make substantive claims as to how the Hodgkin's patient compared, although at that place were no obvious differences in the metabolic profiles of control samples taken at early compared to subsequently stages of lactation.
We recognize that the main limitation of the report is its single instance written report content of a patient undergoing chemotherapy. However, the findings were revealing. Of notation, even with the illness, her milk microbiota earlier treatment was similar to that of good for you lactating women and merely after intervention did the microbiota patterns alter. It has been reported by Hunt et al.[40] that the milk microbiota of a specific private is stable over time, consistent with our study from the good for you sample nerveless four months apart. We believe that the changes in the microbiota are a result of therapeutic agents and non specific to just one patient.
Conclusions
Bacterial and metabolic compositions in human milk, and so disquisitional for amnesty and baby development, tin can change significantly after maternal exposure to chemotherapeutic agents. Further larger cohort studies are warranted to examine microbiota and metabolomic changes associated with chemotherapy and other medications prescribed to lactating mothers and the consequences for the microbiome, the metabolome and long-term health of infants.
Supporting data
The data set supporting the results of this article are included in Boosted files i, 2, three, four, v and 6.
Abbreviations
- DHA:
-
docosahexaenoic acid
- FDR:
-
false discovery rate
- GC-MS:
-
gas chromatography-mass spectrometry
- OTU:
-
operational taxonomic unit
- PCA:
-
principal component assay
- PCR:
-
polymerase chain reaction
- PCoA:
-
chief coordinate analysis
- RDP:
-
Ribosomal Database Project.
References
-
Martin 5, Maldonado-Barragan A, Moles L, Rodriguez-Banos M, Campo RD, Fernandez 50, Rodriguez JM, Jimenez Eastward: Sharing of bacterial strains betwixt breast milk and infant feces. J Hum Lact. 2012, 28: 36-44.
-
Martin R, Langa S, Reviriego C, Jiminez E, Marin ML, Xaus J, Fernandez L, Rodriguez JM: Human milk is a source of lactic acid bacteria for the infant gut. J Pediatr. 2003, 143: 754-758.
-
Stuebe A: The risks of not breastfeeding for mothers and infants. Rev Obstet Gynecol. 2009, 2: 222-231.
-
Silvers KM, Frampton CM, Wickens Yard, Pattemore PK, Ingham T, Fishwick D, Crane J, Town GI, Epton MJ, New Zealand Asthma and Allergy Cohort Study Group: Breastfeeding protects against current asthma upwardly to half dozen years of age. J Pediatr. 2012, 160: 991–6.e1-
-
Guaraldi F, Salvatori G: Effect of breast and formula feeding on gut microbiota shaping in newborns. Front end Jail cell Infect Microbiol. 2012, ii: 94-
-
Chouraqui JP, Grathwohl D, Labaune JM, Hascoet JM, de Montgolfier I, Leclaire K, Giarre M, Steenhout P: Assessment of the safety, tolerance, and protective consequence against diarrhea of babe formulas containing mixtures of probiotics or probiotics and prebiotics in a randomized controlled trial. Am J Clin Nutr. 2008, 87: 1365-1373.
-
Boudry G, David ES, Douard Five, Monteiro IM, Le Huerou-Luron I, Ferraris RP: Role of intestinal transporters in neonatal nutrition: carbohydrates, proteins, lipids, minerals, and vitamins. J Pediatr Gastroenterol Nutr. 2010, 51: 380-401.
-
Kunz C, Rudloff S, Baier W, Klein Due north, Strobel Southward: Oligosaccharides in human milk: structural, functional, and metabolic aspects. Annu Rev Nutr. 2000, 20: 699-722.
-
Agostoni C: Role of long-chain polyunsaturated fatty acids in the first year of life. J Pediatr Gastroenterol Nutr. 2008, 47 (Suppl 2): S41-S44.
-
German JB, Dillard CJ, Ward RE: Bioactive components in milk. Curr Opin Clin Nutr Metab Care. 2002, five: 653-658.
-
Donaldson M, Goodchild JH: Pregnancy, breast-feeding and drugs used in dentistry. J Am Paring Assoc. 2012, 143: 858-871.
-
Drugs and Lactation Database. [http://toxnet.nlm.nih.gov/cgi-bin/sis/htmlgen?LACT]
-
Gartner LM, Morton J, Lawrence RA, Naylor AJ, O'Hare D, Schanler RJ, Eidelman AI, American Academy of Pediatrics Section on Breastfeeding: Breastfeeding and the use of man milk. Pediatrics. 2005, 115: 496-506.
-
Hays KE, Ryu RJ, Swisher EM, Reed E, McManus T, Rybeck B, Petros WP, Hebert MF: Duration of cisplatin excretion in breast milk. J Hum Lact. 2013, 29: 469-472.
-
Pistilli B, Bellettini K, Giovannetti Eastward, Codacci-Pisanelli Chiliad, Azim HA, Benedetti One thousand, Sarno MA, Peccatori FA: Chemotherapy, targeted agents, antiemetics and growth-factors in human milk: how should we counsel cancer patients about breastfeeding?. Cancer Care for Rev. 2013, 39: 207-211.
-
Edgar RC: Search and clustering orders of magnitude faster than BLAST. Bioinformatics. 2010, 26: 2460-2461.
-
Cole JR, Wang Q, Cardenas E, Fish J, Chai B, Farris RJ, Kulam-Syed-Mohideen AS, McGarrell DM, Marsh T, Garrity GM, Tiedje JM: The Ribosomal Database Project: improved alignments and new tools for rRNA analysis. Nucleic Acids Res. 2009, 37 (Database issue): D141-D145.
-
DeSantis TZ, Hugenholtz P, Larsen Due north, Rojas M, Brodie EL, Keller M, Huber T, Dalevi D, Hu P, Andersen GL: Greengenes, a chimera-checked 16S rRNA gene database and workbench compatible with ARB. Appl Environ Microbiol. 2006, 72: 5069-5072.
-
Caporaso JG, Kuczynski J, Stombaugh J, Bittinger K, Bushman FD, Costello EK, Fierer N, Pena AG, Goodrich JK, Gordon JI, Huttley GA, Kelley ST, Knights D, Koenig JE, Ley RE, Lozupone CA, McDonald D, Muegge BD, Pirrung M, Reeder J, Sevinsky JR, Turnbaugh PJ, Walters WA, Widmann J, Yatsunenko T, Zaneveld J, Knight R: QIIME allows assay of loftier-throughput customs sequencing data. Nat Methods. 2010, 7: 335-336.
-
Toll MN, Dehal PS, Arkin AP: FastTree: computing large minimum evolution copse with profiles instead of a distance matrix. Mol Biol Evol. 2009, 26: 1641-1650.
-
Edgar RC: MUSCLE: a multiple sequence alignment method with reduced time and space complexity. BMC Bioinformatics. 2004, v: 113-
-
Lozupone C, Knight R: UniFrac: a new phylogenetic method for comparing microbial communities. Appl Environ Microbiol. 2005, 71: 8228-8235.
-
Colina TC, Walsh KA, Harris JA, Moffett BF: Using ecological diverseness measures with bacterial communities. FEMS Microbiol Ecol. 2003, 43: 1-11.
-
R Evolution Core Team: R: A Linguistic communication and Environment for Statistical Calculating. 2006, Vienna, Austria: R Foundation for Statistical Computing
-
Fernandes Advertizing, Reid J, Macklaim JM, McMurrough TA, Edgell DR, Gloor GB: Unifying the assay of high-throughput sequencing datasets: characterizing RNA-seq, 16S rRNA gene sequencing and selective growth experiments by compositional data analysis. Microbiome. 2014, 2: fifteen-
-
Jaynes ET: Probability Theory: the Logic of Science. 2003, Cambridge, Britain: Cambridge University Press
-
Aitchison J, Egozcue J: Compositional information analysis: Where are we and where should we exist heading?. Math Geol. 2005, 37: 829-850.
-
Stein Due south: An integrated method for spectrum extraction and compound identification from GC/MS data. J Am Soc Mass Spectrom. 1999, ten: 770-781.
-
Styczynski MP, Moxley JF, Tong LV, Walther JL, Jensen KL, Stephanopoulos GN: Systematic identification of conserved metabolites in GC/MS data for metabolomics and biomarker discovery. Anal Chem. 2007, 79: 966-973.
-
Mshvildadze Thou, Neu J, Shuster J, Theriaque D, Li North, Mai V: Intestinal microbial ecology in premature infants assessed with non-culture-based techniques. J Pediatr. 2010, 156: 20-25.
-
Madan JC, Farzan SF, Hibberd PL, Karagas MR: Normal neonatal microbiome variation in relation to environmental factors, infection and allergy. Curr Opin Pediatr. 2012, 24: 753-759.
-
Giongo A, Gano KA, Crabb DB, Mukherjee N, Novelo LL, Casella M, Drew JC, Ilonen J, Knip M, Hyoty H, Veijola R, Simell T, Simell O, Neu J, Wasserfall CH, Schatz D, Atkinson MA, Triplett EW: Toward defining the autoimmune microbiome for blazon 1 diabetes. ISME J. 2011, v: 82-91.
-
Sela DA, Chapman J, Adeuya A, Kim JH, Chen F, Whitehead TR, Lapidus A, Rokhsar DS, Lebrilla CB, German JB, Toll NP, Richardson PM, Mills DA: The genome sequence of Bifidobacterium longum subsp. infantis reveals adaptations for milk utilization within the babe microbiome. Proc Natl Acad Sci U S A. 2008, 105: 18964-18969.
-
Asakuma S, Hatakeyama E, Urashima T, Yoshida Eastward, Katayama T, Yamamoto K, Kumagai H, Ashida H, Hirose J, Kitaoka Grand: Physiology of consumption of human milk oligosaccharides by baby gut-associated bifidobacteria. J Biol Chem. 2011, 286: 34583-34592.
-
Gronlund MM, Gueimonde Yard, Laitinen 1000, Kociubinski G, Gronroos T, Salminen S, Isolauri E: Maternal breast-milk and abdominal bifidobacteria guide the compositional development of the Bifidobacterium microbiota in infants at risk of allergic disease. Clin Exp Allergy. 2007, 37: 1764-1772.
-
Jost T, Lacroix C, Braegger CP, Chassard C: New insights in gut microbiota establishment in healthy breast fed neonates. PLoS One. 2012, 7: e44595-
-
Vael C, Vanheirstraeten L, Desager KN, Goossens H: Denaturing slope gel electrophoresis of neonatal abdominal microbiota in relation to the evolution of asthma. BMC Microbiol. 2011, 11: 68-
-
Vael C, Nelen Five, Verhulst SL, Goossens H, Desager KN: Early intestinal Bacteroides fragilis colonisation and development of asthma. BMC Pulm Med. 2008, 8: xix-
-
Vael C, Verhulst SL, Nelen V, Goossens H, Desager KN: Intestinal microflora and trunk mass alphabetize during the first three years of life: an observational report. Gut Pathog. 2011, iii: 8-
-
Hunt KM, Foster JA, Forney LJ, Schutte UM, Beck DL, Abdo Z, Flim-flam LK, Williams JE, McGuire MK, McGuire MA: Characterization of the diversity and temporal stability of bacterial communities in man milk. PLoS One. 2011, 6: e21313-
-
Ward TL, Hosid S, Ioshikhes I, Altosaar I: Human milk metagenome: a functional chapters assay. BMC Microbiol. 2013, thirteen: 116-
-
Jost T, Lacroix C, Braegger C, Chassard C: Assessment of bacterial diversity in breast milk using culture-dependent and civilization-independent approaches. Br J Nutr. 2013, 110: 1253-1262.
-
Heikkila MP, Saris PE: Inhibition of Staphylococcus aureus by the commensal bacteria of human milk. J Appl Microbiol. 2003, 95: 471-478.
-
Belenguer A, Duncan SH, Calder AG, Holtrop 1000, Louis P, Lobley GE, Flintstone HJ: Two routes of metabolic cross-feeding between Bifidobacterium adolescentis and butyrate-producing anaerobes from the human gut. Appl Environ Microbiol. 2006, 72: 3593-3599.
-
Barcenilla A, Pryde SE, Martin JC, Duncan SH, Stewart CS, Henderson C, Flint HJ: Phylogenetic relationships of butyrate-producing bacteria from the homo gut. Appl Environ Microbiol. 2000, 66: 1654-1661.
-
Duncan SH, Holtrop G, Lobley GE, Calder AG, Stewart CS, Flint HJ: Contribution of acetate to butyrate formation by human faecal bacteria. Br J Nutr. 2004, 91: 915-923.
-
Duncan SH, Louis P, Flintstone HJ: Lactate-utilizing bacteria, isolated from human feces, that produce butyrate as a major fermentation product. Appl Environ Microbiol. 2004, 70: 5810-5817.
-
Hamer HM, Jonkers D, Venema Thou, Vanhoutvin Southward, Troost FJ, Brummer RJ: Review article: the part of butyrate on colonic office. Aliment Pharmacol Ther. 2008, 27: 104-119.
-
Lin HV, Frassetto A, Kowalik EJ, Nawrocki AR, Lu MM, Kosinski JR, Hubert JA, Szeto D, Yao X, Forrest G, Marsh DJ: Butyrate and propionate protect confronting diet-induced obesity and regulate gut hormones via gratuitous fatty acrid receptor 3-contained mechanisms. PLoS One. 2012, 7: e35240-
-
Ramsey MM, Rumbaugh KP, Whiteley M: Metabolite cantankerous-feeding enhances virulence in a model polymicrobial infection. PLoS Pathog. 2011, 7: e1002012-
-
Morse NL: Benefits of docosahexaenoic acid, folic acid, vitamin D and iodine on foetal and infant encephalon development and office post-obit maternal supplementation during pregnancy and lactation. Nutrients. 2012, four: 799-840.
-
Hoffman DR, Boettcher JA, Diersen-Schade DA: Toward optimizing vision and cognition in term infants by dietary docosahexaenoic and arachidonic acrid supplementation: a review of randomized controlled trials. Prostaglandins Leukot Essent Fatty Acids. 2009, 81: 151-158.
-
Yu G, Duchen Thou, Bjorksten B: Fat acid composition in colostrum and mature milk from non-atopic and atopic mothers during the offset vi months of lactation. Acta Paediatr. 1998, 87: 729-736.
-
Duchen Thou, Casas R, Fageras-Bottcher M, Yu Yard, Bjorksten B: Human being milk polyunsaturated long-concatenation fatty acids and secretory immunoglobulin A antibodies and early on childhood allergy. Pediatr Allergy Immunol. 2000, eleven: 29-39.
-
Kok RD, van den Bergh AJ, Heerschap A, Nijland R, van den Berg PP: Metabolic information from the human fetal brain obtained with proton magnetic resonance spectroscopy. Am J Obstet Gynecol. 2001, 185: 1011-1015.
-
Litmanovitz I, Davidson K, Eliakim A, Regev RH, Dolfin T, Arnon South, Bar-Yoseph F, Goren A, Lifshitz Y, Nemet D: Loftier Beta-palmitate formula and bone strength in term infants: a randomized, double-blind, controlled trial. Calcif Tissue Int. 2013, 92: 35-41.
-
Lu P, Bar-Yoseph F, Levi L, Lifshitz Y, Witte-Bouma J, de Bruijn Ac, Korteland-van Male AM, van Goudoever JB, Renes IB: High beta-palmitate fatty controls the intestinal inflammatory response and limits intestinal damage in mucin Muc2 deficient mice. PLoS One. 2013, eight: e65878-
-
Marangoni F, Agostoni C, Lammardo AM, Giovannini Thousand, Galli C, Riva East: Polyunsaturated fatty acid concentrations in human hindmilk are stable throughout 12-months of lactation and provide a sustained intake to the infant during exclusive breastfeeding: an Italian written report. Br J Nutr. 2000, 84: 103-109.
-
Jozwik 1000, Jozwik G, Teng C, Jozwik G, Battaglia FC: Homo breast milk sugars and polyols over the first 10 puerperium days. Am J Hum Biol. 2013, 25: 198-204.
-
Ribeiro Grand, Balcao V, Guimaraes H, Rocha M, Moutinho C, Matos C, Almeida C, Casal Southward, Guerra A: Fat acrid contour of human milk of Portuguese lactating women: prospective study from the 1st to the 16th calendar week of lactation. Ann Nutr Metab. 2008, 53: fifty-56.
-
Lopez-Lopez A, Lopez-Sabater MC, Campoy-Folgoso C, Rivero-Urgell K, Castellote-Bargallo AI: Fatty acid and sn-2 fatty acid composition in human milk from Granada (Spain) and in babe formulas. Eur J Clin Nutr. 2002, 56: 1242-1254.
Acknowledgements
We would like to thank Jean Macklaim for her advice and guidance in analyzing the 16S rRNA sequencing data and Tim McDowell at Agriculture and Agri-Food Canada for technical support with GC-MS. Funding sources to individuals: CU - CIHR Strategic Training Program in Cancer Enquiry and Technology Transfer; and the Translational Breast Cancer Studentship; AM - Ontario Graduate Scholarship. Written informed consent was obtained from the patients for publication of this manuscript and accompanying images.
Writer information
Affiliations
Respective author
Additional information
Competing interests
The authors declare that they have no competing interests.
Authors' contributions
CU designed the study, recruited subjects, nerveless and prepared the samples for microbial analysis, analyzed 16S rRNA sequencing data and wrote the manuscript. AM performed GC-MS analysis on the milk samples and reviewed the manuscript. MA helped with study blueprint and recruitment and reviewed the manuscript. JB provided input into study design and reviewed the manuscript. MS contributed essentially to the pattern and conquering of the metabolomic data, analysis and manuscript review. GG provided co-supervision, teaching and input into microbiome acquisition and analysis and manuscript review. GR conceptualized the study, helped with study design and manuscript writing, supervised data collection and analysis and provided financial back up. All authors read and canonical the last manuscript.
Electronic supplementary material
40168_2014_53_MOESM1_ESM.xlsx
Boosted file 1: Tabular array S1: Summary of taxonomic results and full-length V6 16S rRNA sequence of each OTU. (XLSX 33 KB)
Boosted file 2: Table S2: Summary of clinical information. (XLSX 10 KB)
40168_2014_53_MOESM3_ESM.dr.
Boosted file iii: Table S3: Comparing of relative abundances of different genera detected in milk between the chemotherapy and non-treatment groups. Values in the 2nd and third columns represent the base ii logarithm of the median affluence in all samples within a group (that is, Wk0/H samples (non-treatment group) or Wk4-16 samples (chemotherapy group) relative to the geometric hateful abundance, which has a value of 0. Thus, positive values are college than the geometric mean and are thus more abundant than negative values, which are lower than the geometric hateful. Significant differences were based on FDR values of <0.1. Out of the 49 genera identified, 22 were significantly different between the 2 groups. (Physician 102 KB)
Additional file 4: Table S4: Taxa present in every sample within a group. (XLSX 45 KB)
40168_2014_53_MOESM5_ESM.xlsx
Boosted file five: Table S5: Relative abundances of metabolites identified in breast milk by GC-MS. Relative abundance values were constructed by the Spectconnect program, which assigns the sample with the greatest concentration (elevation area) of each metabolite an arbitrary value. All other samples are so fabricated proportional to this value according to peak area. Values represent an average of ii technical replicates. *Metabolite identity confirmed by accurate standards. The metabolites referred to with numbers in the first column stand for un-annotated metabolites. The P values between the week 0 and chemotherapy groups were calculated using an unpaired t-test with Bonferroni correction. (XLSX 38 KB)
40168_2014_53_MOESM6_ESM.pdf
Additional file six: Figure S1: Comparison of relative abundance of metabolites in milk between week 0 and chemotherapy. The relative abundances of DHA, inositol and palmitic acid are shown above, with each point on the graph representing a different milk sample. DHA and inositol were significantly decreased past chemotherapy (unpaired t-test with Bonferroni correction, P < 0.05). While palmitic acid was also decreased by chemotherapy, these results were not statistically pregnant. Values represent an average of ii technical replicates. (PDF 53 KB)
Rights and permissions
Open Admission This article is licensed under a Creative Commons Attribution 4.0 International License, which permits utilise, sharing, adaptation, distribution and reproduction in whatsoever medium or format, as long as you give advisable credit to the original author(s) and the source, provide a link to the Creative Commons licence, and betoken if changes were fabricated.
The images or other third party material in this article are included in the article's Creative Commons licence, unless indicated otherwise in a credit line to the cloth. If material is non included in the article'south Creative Commons licence and your intended utilize is not permitted by statutory regulation or exceeds the permitted use, you will need to obtain permission directly from the copyright holder.
To view a copy of this licence, visit https://creativecommons.org/licenses/by/4.0/.
The Artistic Eatables Public Domain Dedication waiver (https://creativecommons.org/publicdomain/zero/1.0/) applies to the data fabricated bachelor in this article, unless otherwise stated in a credit line to the data.
Reprints and Permissions
Virtually this article
Cite this article
Urbaniak, C., McMillan, A., Angelini, M. et al. Effect of chemotherapy on the microbiota and metabolome of human milk, a instance written report. Microbiome 2, 24 (2014). https://doi.org/10.1186/2049-2618-2-24
-
Received:
-
Accepted:
-
Published:
-
DOI : https://doi.org/ten.1186/2049-2618-2-24
Keywords
- 16S rRNA factor sequencing
- Homo milk microbiome
- Metabolome
Source: https://microbiomejournal.biomedcentral.com/articles/10.1186/2049-2618-2-24